Automatic clustering of both source web pages as well as web search
results that returned from Search Engine for a topic is one of the
most important and efficient techniques to help end users in navigation,
discrimination, summarization and interpretation of the Web. Most
exiting clustering approaches that based on common terms sharing
cannot adapt well for web page clustering in a lot of cases due
to special features of web data. We proposed a novel contents-link
coupled clustering approach by combining contents (terms appeared
in snippet, title, meta-contents and anchor window of in-links)
analysis and link analysis (co-citation and coupling analysis) to
offer a much better solution for web page clustering both in terms
of effectiveness and topic discrimination.
We applied the proposed clustering approach to search results of
various topics and got positive and interesting results. Based on
term vector, we attach each cluster with tagging terms to give end
users the topic of each cluster from semantic point of view. E.g.
for topic "jaguar", we could obtain clusters talking about
car, animal, game, driver club、magazine etc. In particular, we study
in detail about the respective contributions of term, out-link and
in-link on clustering procedure and in turn on how to effectively
combine the two very different features (term and link) into one
clustering schema to make the proposed contents-link coupled clustering
adapt well to various kinds of topic for robust clustering.
(Wang Yitong)
|
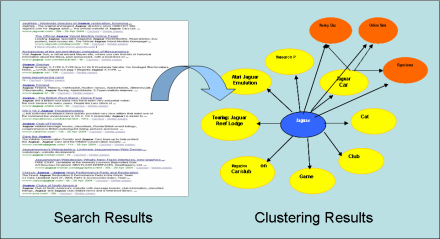 |